Llama 4 family of models from Meta are now available in SageMaker JumpStart
Today, we’re excited to announce the availability of Llama 4 Scout and Maverick models in Amazon SageMaker JumpStart. In this blog post, we walk you through how to deploy and prompt a Llama-4-Scout-17B-16E-Instruct model using SageMaker JumpStart.
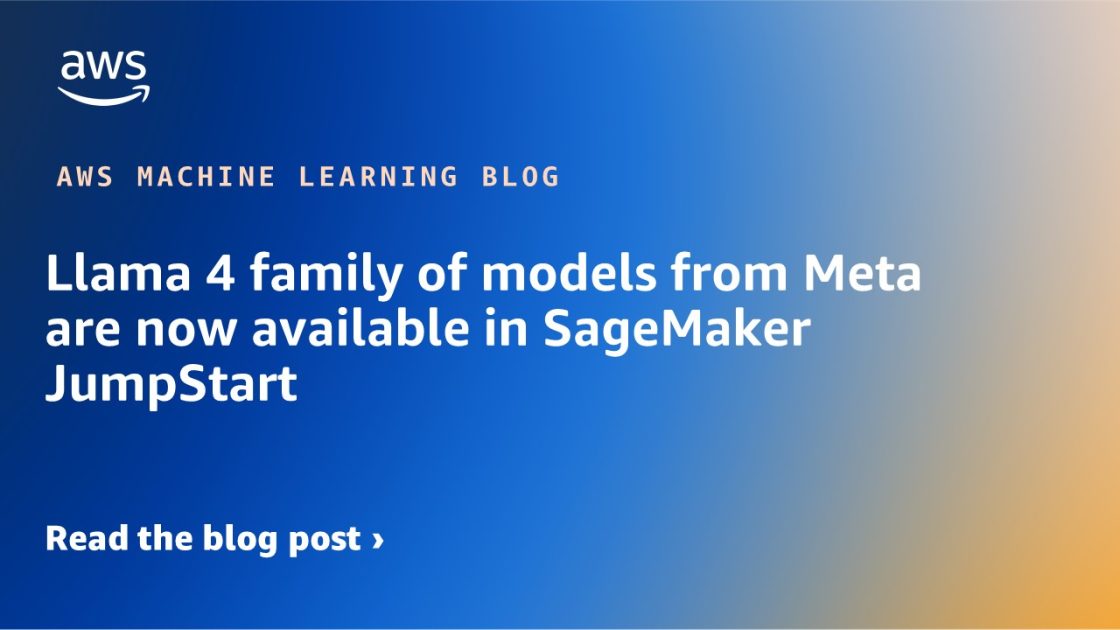
Today, we’re excited to announce the availability of Llama 4 Scout and Maverick models in Amazon SageMaker JumpStart and coming soon in Amazon Bedrock. Llama 4 represents Meta’s most advanced multimodal models to date, featuring a mixture of experts (MoE) architecture and context window support up to 10 million tokens. With native multimodality and early fusion technology, Meta states that these new models demonstrate unprecedented performance across text and vision tasks while maintaining efficient compute requirements. With a dramatic increase on supported context length from 128K in Llama 3, Llama 4 is now suitable for multi-document summarization, parsing extensive user activity for personalized tasks, and reasoning over extensive codebases. You can now deploy the Llama-4-Scout-17B-16E-Instruct, Llama-4-Maverick-17B-128E-Instruct, and Llama-4-Maverick-17B-128E-Instruct-FP8 models using SageMaker JumpStart in the US East (N. Virginia) AWS Region.
In this blog post, we walk you through how to deploy and prompt a Llama-4-Scout-17B-16E-Instruct model using SageMaker JumpStart.
Llama 4 overview
Meta announced Llama 4 today, introducing three distinct model variants: Scout, which offers advanced multimodal capabilities and a 10M token context window; Maverick, a cost-effective solution with a 128K context window; and Behemoth, in preview. These models are optimized for multimodal reasoning, multilingual tasks, coding, tool-calling, and powering agentic systems.
Llama 4 Maverick is a powerful general-purpose model with 17 billion active parameters, 128 experts, and 400 billion total parameters, and optimized for high-quality general assistant and chat use cases. Additionally, Llama 4 Maverick is available with base and instruct models in both a quantized version (FP8) for efficient deployment on the Instruct model and a non-quantized (BF16) version for maximum accuracy.
Llama 4 Scout, the more compact and smaller model, has 17 billion active parameters, 16 experts, and 109 billion total parameters, and features an industry-leading 10M token context window. These models are designed for industry-leading performance in image and text understanding with support for 12 languages, enabling the creation of AI applications that bridge language barriers.
See Meta’s community license agreement for usage terms and more details.
SageMaker JumpStart overview
SageMaker JumpStart offers access to a broad selection of publicly available foundation models (FMs). These pre-trained models serve as powerful starting points that can be deeply customized to address specific use cases. You can use state-of-the-art model architectures—such as language models, computer vision models, and more—without having to build them from scratch.
With SageMaker JumpStart, you can deploy models in a secure environment. The models can be provisioned on dedicated SageMaker inference instances can be isolated within your virtual private cloud (VPC). After deploying an FM, you can further customize and fine-tune it using the extensive capabilities of Amazon SageMaker AI, including SageMaker inference for deploying models and container logs for improved observability. With SageMaker AI, you can streamline the entire model deployment process.
Prerequisites
To try the Llama 4 models in SageMaker JumpStart, you need the following prerequisites:
- An AWS account that will contain all your AWS resources.
- An AWS Identity and Access Management (IAM) role to access SageMaker AI. To learn more about how IAM works with SageMaker AI, see Identity and Access Management for Amazon SageMaker AI.
- Access to Amazon SageMaker Studio and a SageMaker AI notebook instance or an interactive development environment (IDE) such as PyCharm or Visual Studio Code. We recommend using SageMaker Studio for straightforward deployment and inference.
- Access to accelerated instances (GPUs) for hosting the LLMs.
Discover Llama 4 models in SageMaker JumpStart
SageMaker JumpStart provides FMs through two primary interfaces: SageMaker Studio and the Amazon SageMaker Python SDK. This provides multiple options to discover and use hundreds of models for your specific use case.
SageMaker Studio is a comprehensive integrated development environment (IDE) that offers a unified, web-based interface for performing all aspects of the AI development lifecycle. From preparing data to building, training, and deploying models, SageMaker Studio provides purpose-built tools to streamline the entire process.
In SageMaker Studio, you can access SageMaker JumpStart to discover and explore the extensive catalog of FMs available for deployment to inference capabilities on SageMaker Inference. You can access SageMaker JumpStart by choosing JumpStart in the navigation pane or by choosing JumpStart from the Home page in SageMaker Studio, as shown in the following figure.
Alternatively, you can use the SageMaker Python SDK to programmatically access and use SageMaker JumpStart models. This approach allows for greater flexibility and integration with existing AI and machine learning (AI/ML) workflows and pipelines.
By providing multiple access points, SageMaker JumpStart helps you seamlessly incorporate pre-trained models into your AI/ML development efforts, regardless of your preferred interface or workflow.
Deploy Llama 4 models for inference through the SageMaker JumpStart UI
On the SageMaker JumpStart landing page, you can find all the public pre-trained models offered by SageMaker AI. You can then choose the Meta model provider tab to discover all the available Meta models.
If you’re using SageMaker Classic Studio and don’t see the Llama 4 models, update your SageMaker Studio version by shutting down and restarting. For more information about version updates, see Shut down and Update Studio Classic Apps.
- Search for Meta to view the Meta model card. Each model card shows key information, including:
- Model name
- Provider name
- Task category (for example, Text Generation)
- Select the model card to view the model details page.
The model details page includes the following information:
- The model name and provider information
- Deploy button to deploy the model
- About and Notebooks tabs with detailed information
The About tab includes important details, such as:
- Model description
- License information
- Technical specifications
- Usage guidelines
Before you deploy the model, we recommended you review the model details and license terms to confirm compatibility with your use case.
- Choose Deploy to proceed with deployment.
- For Endpoint name, use the automatically generated name or enter a custom one.
- For Instance type, use the default: p5.48xlarge.
- For Initial instance count, enter the number of instances (default: 1).
Selecting appropriate instance types and counts is crucial for cost and performance optimization. Monitor your deployment to adjust these settings as needed. - Under Inference type, Real-time inference is selected by default. This is optimized for sustained traffic and low latency.
- Review all configurations for accuracy. For this model, we strongly recommend adhering to SageMaker JumpStart default settings and making sure that network isolation remains in place.
- Choose Deploy. The deployment process can take several minutes to complete.
When deployment is complete, your endpoint status will change to InService. At this point, the model is ready to accept inference requests through the endpoint. You can monitor the deployment progress on the SageMaker console Endpoints page, which will display relevant metrics and status information. When the deployment is complete, you can invoke the model using a SageMaker runtime client and integrate it with your applications.
Deploy Llama 4 models for inference using the SageMaker Python SDK
When you choose Deploy and accept the terms, model deployment will start. Alternatively, you can deploy through the example notebook by choosing Open Notebook. The notebook provides end-to-end guidance on how to deploy the model for inference and clean up resources.
To deploy using a notebook, start by selecting an appropriate model, specified by the model_id
. You can deploy any of the selected models on SageMaker AI.
You can deploy the Llama 4 Scout model using SageMaker JumpStart with the following SageMaker Python SDK code:
This deploys the model on SageMaker AI with default configurations, including default instance type and default VPC configurations. You can change these configurations by specifying non-default values in JumpStartModel. To successfully deploy the model, you must manually set accept_eula=True
as a deploy method argument. After it’s deployed, you can run inference against the deployed endpoint through the SageMaker predictor:
Recommended instances and benchmark
The following table lists all the Llama 4 models available in SageMaker JumpStart along with the model_id, default instance types, and the maximum number of total tokens (sum of number of input tokens and number of generated tokens) supported for each of these models. For increased context length, you can modify the default instance type in the SageMaker JumpStart UI.
Model name | Model ID | Default instance type | Supported instance types |
Llama-4-Scout-17B-16E-Instruct | meta-vlm-llama-4-scout-17b-16e-instruct |
ml.p5.48xlarge | ml.g6e.48xlarge, ml.p5.48xlarge, ml.p5en.48xlarge |
Llama-4-Maverick-17B-128E-Instruct | meta-vlm-llama-4-maverick-17b-128e-instruct |
ml.p5.48xlarge | ml.p5.48xlarge, ml.p5en.48xlarge |
Llama 4-Maverick-17B-128E-Instruct-FP8 | meta-vlm-llama-4-maverick-17b-128-instruct-fp8 |
ml.p5.48xlarge | ml.p5.48xlarge, ml.p5en.48xlarge |
Inference and example prompts for Llama 4 Scout 17B 16 Experts model
You can use the Llama 4 Scout model for text and image or vision reasoning use cases. With that model, you can perform a variety of tasks, such as image captioning, image text retrieval, visual question answering and reasoning, document visual question answering, and more.
In the following sections we show example payloads, invocations, and responses for Llama 4 Scout that you can use against your Llama 4 model deployments using Sagemaker JumpStart.
Text-only input
Input:
Response:
Single-image input
In this section, let’s test Llama 4’s multimodal capabilities. By merging text and vision tokens into a unified processing backbone, Llama 4 can seamlessly understand and respond to queries about an image. The following is an example of how you can prompt Llama 4 to answer questions about an image such as the one in the example:
Image:
Input:
Response:
The Llama 4 model on JumpStart can take in the image provided via a URL, underlining its powerful potential for real-time multimodal applications.
Multi-image input
Building on its advanced multimodal functionality, Llama 4 can effortlessly process multiple images at the same time. In this demonstration, the model is prompted with two image URLs and tasked with describing each image and explaining their relationship, showcasing its capacity to synthesize information across several visual inputs. Let’s test this below by passing in the URLs of the following images in the payload.
Image 1:
Image 2:
Input:
Response:
As you can see, Llama 4 excels in handling multiple images simultaneously, providing detailed and contextually relevant insights that emphasize its robust multimodal processing abilities.
Codebase analysis with Llama 4
Using Llama 4 Scout’s industry-leading context window, this section showcases its ability to deeply analyze expansive codebases. The example extracts and contextualizes the buildspec-1-10-2.yml
file from the AWS Deep Learning Containers GitHub repository, illustrating how the model synthesizes information across an entire repository. We used a tool to ingest the whole repository into plaintext that we provided to the model as context:
Input:
Output:
Multi-document processing
Harnessing the same extensive token context window, Llama 4 Scout excels in multi-document processing. In this example, the model extracts key financial metrics from Amazon 10-K reports (2017-2024), demonstrating its capability to integrate and analyze data spanning multiple years—all without the need for additional processing tools.
Input:
Output:
Clean up
To avoid incurring unnecessary costs, when you’re done, delete the SageMaker endpoints using the following code snippets:
Alternatively, using the SageMaker console, complete the following steps:
- On the SageMaker console, under Inference in the navigation pane, choose Endpoints.
- Search for the embedding and text generation endpoints.
- On the endpoint details page, choose Delete.
- Choose Delete again to confirm.
Conclusion
In this post, we explored how SageMaker JumpStart empowers data scientists and ML engineers to discover, access, and deploy a wide range of pre-trained FMs for inference, including Meta’s most advanced and capable models to date. Get started with SageMaker JumpStart and Llama 4 models today.
For more information about SageMaker JumpStart, see Train, deploy, and evaluate pretrained models with SageMaker JumpStart and Getting started with Amazon SageMaker JumpStart.
About the authors
Marco Punio is a Sr. Specialist Solutions Architect focused on generative AI strategy, applied AI solutions, and conducting research to help customers hyper-scale on AWS. As a member of the Third-party Model Provider Applied Sciences Solutions Architecture team at AWS, he is a global lead for the Meta–AWS Partnership and technical strategy. Based in Seattle, Washington, Marco enjoys writing, reading, exercising, and building applications in his free time.
Chakravarthy Nagarajan is a Principal Solutions Architect specializing in machine learning, big data, and high performance computing. In his current role, he helps customers solve real-world, complex business problems using machine learning and generative AI solutions.
Banu Nagasundaram leads product, engineering, and strategic partnerships for Amazon SageMaker JumpStart, the SageMaker machine learning and generative AI hub. She is passionate about building solutions that help customers accelerate their AI journey and unlock business value.
Malav Shastri is a Software Development Engineer at AWS, where he works on the Amazon SageMaker JumpStart and Amazon Bedrock teams. His role focuses on enabling customers to take advantage of state-of-the-art open source and proprietary foundation models and traditional machine learning algorithms. Malav holds a Master’s degree in Computer Science.
Niithiyn Vijeaswaran is a Generative AI Specialist Solutions Architect with the Third-party Model Science team at AWS. His area of focus is AWS AI accelerators (AWS Neuron). He holds a Bachelor’s degree in Computer Science and Bioinformatics.
Baladithya Balamurugan is a Solutions Architect at AWS focused on ML deployments for inference and using AWS Neuron to accelerate training and inference. He works with customers to enable and accelerate their ML deployments on services such as Amazon Sagemaker and Amazon EC2. Based in San Francisco, Baladithya enjoys tinkering, developing applications, and his home lab in his free time.
John Liu has 14 years of experience as a product executive and 10 years of experience as a portfolio manager. At AWS, John is a Principal Product Manager for Amazon Bedrock. Previously, he was the Head of Product for AWS Web3 and Blockchain. Prior to AWS, John held various product leadership roles at public blockchain protocols and fintech companies, and also spent 9 years as a portfolio manager at various hedge funds.